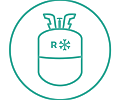
Summary
It’s very difficult to design a completely sealed chiller system, so refrigerant leakage is almost the most common fault in a positive pressure cycle. When a refrigerant leak occurs, chillers will have higher power consumption, even causing health and safety accidents in a closed environment. Leaking refrigerants with high global warming potential (GWP) will accelerate the greenhouse effect. This study presents a semi-supervised machine learning approach to detect refrigerant leakageand all data used for detecting leakage are from pre-installed sensors. A sophisticated experimental method was designed to collect data from a centrifugal chiller and the algorithm of anomaly detection using long short-term memory (LSTM-AD)is discussed with reconstruction error. The LSTMencoder and decoder models are trained on normal data and is used to detect leakage. It’sverified that detection sensitivity can reach 6% and the best detection coverage for leakage 6%, 11% and 16% are respectively 66%, 95% and 95%.
Available documents
Format PDF
Pages: 8 p.
Available
Public price
20 €
Member price*
Free
* Best rate depending on membership category (see the detailed benefits of individual and corporate memberships).
Details
- Original title: A semi-supervised data-driven approach for chiller refrigerant leakage detection.
- Record ID : 30031015
- Languages: English
- Subject: Technology
- Source: 3rd IIR conference on HFO Refrigerants and low GWP Blends. Shanghai, China.
- Publication date: 2023/04/05
- DOI: http://dx.doi.org/10.18462/iir.HFO2023.0005
Links
See other articles from the proceedings (28)
See the conference proceedings
Indexing
-
Development of a remote refrigerant leakage det...
- Author(s) : KIMURA S., MORIWAKI M., YOSHIMI M., YAMADA S., HIKAWA T., KASAHARA S.
- Date : 2022/07/10
- Languages : English
- Source: 2022 Purdue Conferences. 19th International Refrigeration and Air-Conditioning Conference at Purdue.
- Formats : PDF
View record
-
Integration of dynamic model and classification...
- Author(s) : AGUILERA J. J., MEESENBURG W., SCHULTE A., OMMEN T., MARKUSSEN W. B., ZÜHLSDORF B., POULSEN J. L., FÖRSTERLING S., ELMEGAARD B.
- Date : 2022/06/13
- Languages : English
- Source: 15th IIR-Gustav Lorentzen Conference on Natural Refrigerants (GL2022). Proceedings. Trondheim, Norway, June 13-15th 2022.
- Formats : PDF
View record
-
A comprehensive review: Fault detection, diagno...
- Author(s) : SINGH V., MATHUR J., BHATIA A.
- Date : 2022/12
- Languages : English
- Source: International Journal of Refrigeration - Revue Internationale du Froid - vol. 144
- Formats : PDF
View record
-
A robust fault diagnosis method for HVAC system...
- Author(s) : ZHU X., CHEN S., CHEN K., LIANG X., REN T., JIN X., DU Z.
- Date : 2023/08/21
- Languages : English
- Source: Proceedings of the 26th IIR International Congress of Refrigeration: Paris , France, August 21-25, 2023.
- Formats : PDF
View record
-
An interpretable machine learning method for fa...
- Author(s) : CHEN K., ZHU X., CHEN S., DU Z.
- Date : 2023/08/21
- Languages : English
- Source: Proceedings of the 26th IIR International Congress of Refrigeration: Paris , France, August 21-25, 2023.
- Formats : PDF
View record